Image Commercially Licensed From: Unsplash
By: Navin Kamuni
Amidst the deluge of data in the modern era, time series analysis has become indispensable in a wide range of fields, from climatic forecasting to stock market trend prediction. Fundamentally, time series analysis is the process of interpreting, modeling, and forecasting data points that are gathered one after the other over a period of time. This field has undergone a revolution with the introduction of machine learning (ML), which provides hitherto unseen insights and predictive power. However, incorporating machine learning into time series analysis is a difficult undertaking that presents a number of unique, intriguing, and complicated challenges.
Machine learning appears to be a natural ally for time series analysis because of its capacity to learn from and make predictions based on data. When these fields work together, deeper insights into temporal data patterns that were previously hidden or too complex to understand could be unlocked. However, due to the very characteristics of time series data, including their sequential structure, potential non-stationarity, and the complexities of temporal correlations, getting these insights is fraught with difficulties.
There are numerous difficulties. Every step of applying machine learning to this domain, from guaranteeing the quantity and quality of data to capturing the minute temporal dependencies that characterize time series, calls for specific methods and careful thought. Another layer of complexity is added by the dynamism of time series data, where patterns can change and evolve over time. Models that held true a day ago might not hold true today, requiring a continuous evolution of approaches and techniques.
It’s important to recognize the difficulty of the task at hand and the creativity needed to traverse this challenging terrain as we set out on this exploration. Applying machine learning to time series analysis presents both opportunities and challenges. It’s not just a technical undertaking; it’s a journey into a realm where data tells the story of time and where the patterns of the past write the future.
Challenge 1: Data Quality and Quantity
- Handling Missing Values: In contrast to other types of data, time series data frequently has gaps or missing values because of a variety of problems, such as broken sensors or poor data transmission. It is imperative to develop robust imputation techniques.
- Volume of Data: Processing and storage become difficult due to the sheer amount of data, particularly in high-frequency time series (such as stock market data). Effective ML application requires effective data handling techniques.
Challenge 2: Temporal Dependencies and Seasonality
- Seizing Seasonality: Seasonality is present in a lot of time series. For ML models to produce reliable predictions, these cyclical variations must be taken into account.
- Dealing with Stationarity The process of machine learning modeling is made more difficult by non-stationary data, whose statistical characteristics vary over time. It is frequently necessary to use techniques like transformation or differencing.
Challenge 3: Feature Engineering and Selection
Time-based Features: Using date and time components (holidays, day of the week, etc.) can have a big impact on model performance beyond just basic features.
High-Dimensional Feature Space: Time series data may produce a feature space that is highly dimensional, which raises the computational and complexity requirements for machine learning models.
Challenge 4: Model Complexity and Interpretability
Finding a Balance Between Complexity and Performance: While highly complex models may perform well, they may also require more computational power and be harder to understand.
Explaining Predictions: Transparency and explainability of model predictions are just as crucial in crucial domains like healthcare as accuracy.
Challenge 5: Overfitting and Model Evaluation
- Evaluation Metrics: Selecting relevant metrics that accurately capture the time series forecasting model’s performance is crucial.
- Dynamic Environments: Time series data must be able to adapt and not just fit historical data because these environments, such as stock markets, are dynamic and subject to rapid change.
Challenge 6: Real-time Analysis, Scalability, and Deployment
- Stream Processing: ML models need to efficiently process and analyze data streams in scenarios such as IoT and real-time monitoring.
- Deployment Challenges: Efficient infrastructure and ongoing performance degradation monitoring are necessary when deploying machine learning models in a production setting, especially for real-time applications.
Conclusion:
The process of integrating machine learning with time series analysis involves a number of unique obstacles that call for specialized answers. More reliable, understandable, and effective machine learning models that are skilled at handling the subtleties of time series data will be the field’s future. There is a limitless potential for innovative applications and advancements in this field as science and technology develop.
References:
- Data Quality and Quantity: Kaggle Competitions: Machine Learning Time Series Forecasting Problems (machinelearningmastery.com)
- Temporal Dependencies and Seasonality: Temporal Fusion Transformer (TFT): Google Research Blog on Temporal Fusion Transformers (blog.research.google)
- Feature Engineering and Selection: Time-Series Forecasting in Data Center Telemetry: Challenges and Approaches to Time-Series Forecasting in Data Center Telemetry: A Survey (ar5iv.org)
- Model Complexity and Interpretability: Healthcare Time Series Analysis: Time Series in Healthcare: Challenges and Solutions (vanderschaar-lab.com)
——————————————————————————————————————————————
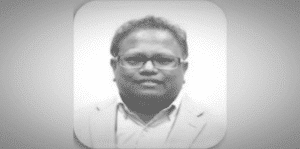
Sourced Photo
About the Author – Navin Kamuni:
An AI Visionary and Technology
Innovator
Navin Kamuni stands as a notable figure in the realm of contact center technologies and AI innovation. With a career spanning over two decades, he has been instrumental in driving technological advancements in the industry. His expertise extends to the intricate domains of Artificial Intelligence (AI) and Machine Learning (ML), areas where he has demonstrated considerable proficiency and forward-thinking.
Beyond his professional achievements, Kamuni is a revered mentor and guide within the tech community. As an author, Kamuni has ventured into the literary world with his book, “Connect.AI: The New Era of Digital Experiences.” The book encapsulates his deep understanding of AI’s role in transforming digital customer experiences, positioning him as a thought leader in the field.
Kamuni’s contributions extend to writing insightful articles and research papers, and his participation in conferences and seminars has made him a respected voice in the tech community. His commitment to sharing knowledge and inspiring others is evident in his active engagement with various platforms, including IEEE and Medium.com.
For those interested in learning more about Navin Kamuni’s professional journey, achievements, and current projects, his LinkedIn profile offers a comprehensive overview. Connect with him on LinkedIn to explore his extensive experience, publications, and contributions to the field of technology and AI.
In summary, Navin Kamuni is a visionary leader whose work at the intersection of technology and AI continues to inspire and influence the industry’s landscape.